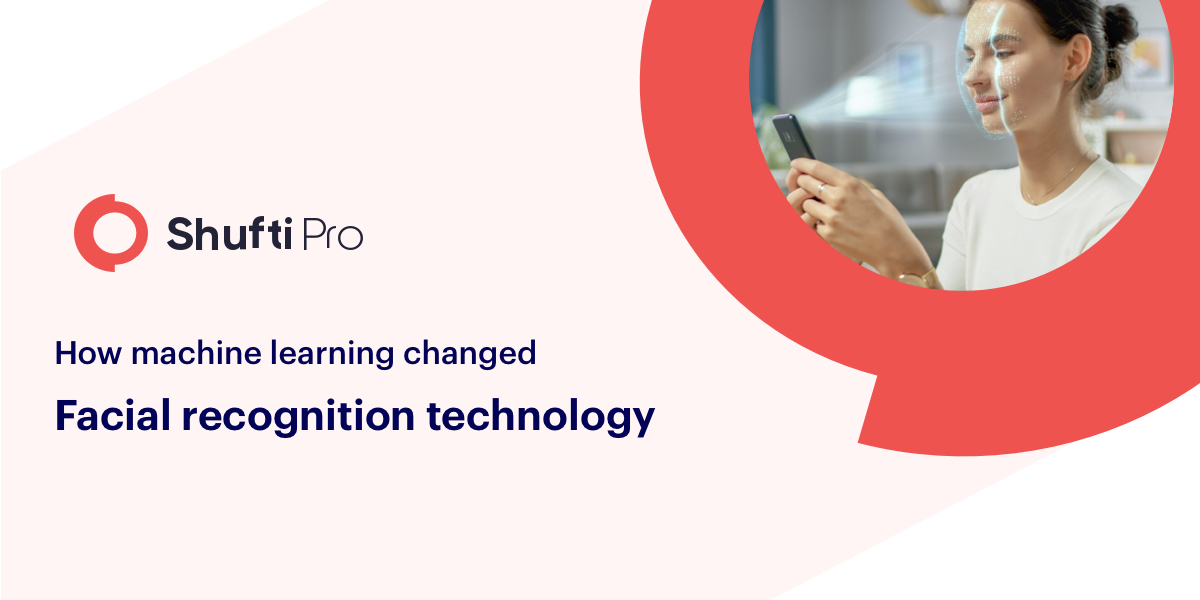
How machine learning changed facial recognition technology?
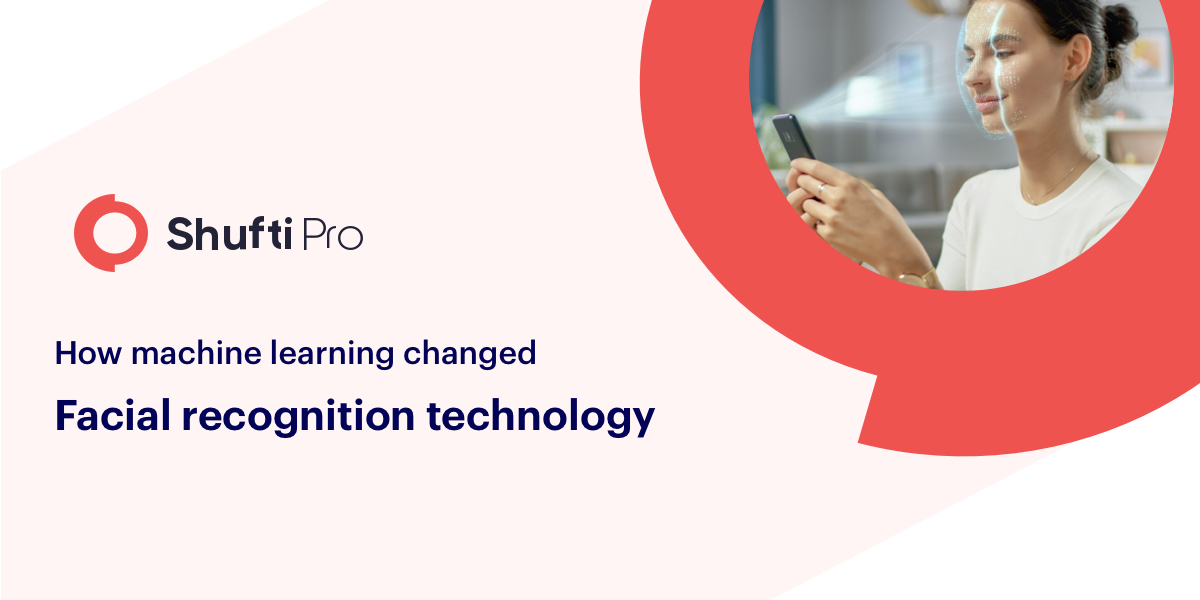
BEFORE YOU GO...
Check how Shufti Pro can verify your customers within seconds
Request DemoNo thanks
We are entering a new era of fast and secure authentication clubbed with a perfect storm of digital transformation. The convergence of AI and biometrics is a part of this transformation and as with many technological breakthroughs, this transformation is not down to the advancement in a single technology.
The implementation of face recognition has seen many iterations starting from its origin in the 1960s when it was manually implemented with a RAND tablet (a graphical computational device). The technology was incrementally refined during the last century. However, adoption of facial recognition on a large scale became possible, thanks to the breakthrough of deep machine learning in the early 2010s. In this article, we will try to elaborate how machine learning has changed facial recognition technology and the impact it has on the development of robust authentication systems.
Facial recognition is a way to recognize a person’s face using technology. A face recognition system uses facial biometrics to map facial features using an image or a picture from a video frame. It compares the information from a database to find the exact match. Face recognition helps verify someone’s identity and is a widely used security measure.
The facial recognition market is expected to grow to $7.7 billion by 2022 and it’s because the technology has all kinds of commercial applications. From airport security to healthcare and customer authentication, face recognition is now widely adopted around the globe.
Deep machine learning or deep neural networks are about a computer program that learns on its own. The fact that it is called “neural” or “neural network” comes from the basis that the technology is inspired by the human brain’s properties to transform data into information. It is a variant of the more general concept of machine learning, which in turn is part of a more comprehensive concept called artificial intelligence.
With deep machine learning, an algorithm serves training data and delivers results. But in between input and output, the algorithm interprets the signals – i.e (training data) – in a number of layers. For each new layer, the degree of abstraction increases.
Say, you want to build a deep neural network that can differentiate different faces or that can determine which faces are identical. Training data should then be a large number of images of the faces. The larger the dataset, the more accurate the network, at least in theory.
A computer does not “see” a face in the image, but several values representing different pixels. With the pixels as a background, the deep neural network learns to find patterns. For each layer passed in the network, some patterns become more interesting (stronger signal between the “neurons” in the network) while others are nonchalant (weaker signal). During training, the “weights” of the various signals are varied to produce the desired result better and better.
The first, second and hundredth time the algorithm performs this procedure, the results are usually not as good, but eventually, the network can achieve impressive results. In a way, one can say that the network has learned to abstract and generalize, from raw pixel values to the classification of different people’s faces.
But that is perhaps not what we humans think of when we use terms such as generalization; it is rather the network has worked out some metrics that are unique to each face. If the pre-trained network is served a new image on a face, the network can match its measurement values to the faces on other images. If the network generates roughly the same values for different images, it is likely the same person on both images.
It is called deep machine learning because such a model can use multiple – sometimes a hundred layers. This is symbolic; humans cannot understand how the computer program finds patterns. It operates in different layers.
Although the algorithms are developed and refined as they are, there are two other reasons behind the breakthrough of the deep neural networks: access to large datasets and cheap computing power, especially in the form of graphics cards that were most often associated with computer games.
It may also be borne in mind that the method described above for classification purposes is only one of many but is commonly used.
While converging machine learning algorithms with face recognition makes it more accurate and fast, there is another feature that makes machine learning a must-have for face authentication – Anti Spoofing. This innovative technology shows a lot of promise and has the potential to revolutionize the way we access sensitive information.
Even though face recognition is promising, it does have some flaws. Simple face recognition systems could easily be spoofed by using paper-based images from the internet. These spoof attacks could lead to a leak of sensitive data. This is where the need for anti-spoofing systems come into play. Facial anti-spoofing techniques help prevent fraud, reduce theft and protect sensitive information.
Presentation attacks are the most common spoofing attacks used to fool facial recognition systems. The presentation attacks are aimed to subvert the face authentication software by presenting a facial artefact. Popular face artefacts include printed photos, replaying the video, 2D and 3D facial masks.
AI-based anti-spoofing technology has the ability to detect and combat facial spoofing attacks. With features like 3D depth perception, liveness detection, and microexpression analysis, our deep learning based facial authentication system could accurately analyze the facial data and identify almost all kinds of spoofing attacks. Shufti Pro detected 42 different spoof attacks in 2019. Among these 3D face mask attacks were in high volumes – almost 30%.
Machine learning-based presentation attack detection algorithms are used to automatically identify these artefacts to improve the security and reliability of biometric authentication systems.
Machine learning-based face verification systems rely on 3D liveness detection feature for successful detection of spoofing attacks including 3D photo masking, deep fakes, face morphing attacks, forgery, and photoshopped images. Liveness detection verifies whether a user is present or is using a photo to spoof the system.
The human face has already become a perfect means of authentication and will have more impact on the digital transformation in the future. By using the face as an identifier, we are already able to open an online account, make online payments, unlock the smartphones, go through checking control at the airport or access medical history in the healthcare sector.
In general, facial biometric technology has widespread potential in four categories: law enforcement and security, online marketing and retail sector, health sector, and social media platforms. AI-empowered face recognition technology has the potential to become predominant in the future.
One of the future implications of technology is identifying facial expressions. Detecting emotions with the help of technology is a difficult task but machine learning algorithms have the promising potential to automate this task. By recognizing facial expressions, businesses will be able to process images and videos in real-time for better monitoring and predictions hence saving the cost and time.
Although it’s hard to predict the future facial recognition technology with the rapid growth and adoption of technology, it will become more widely adopted across the globe with more sophisticated features.