AI-Powered OCR to replace data entry in 2020 – A detailed insight
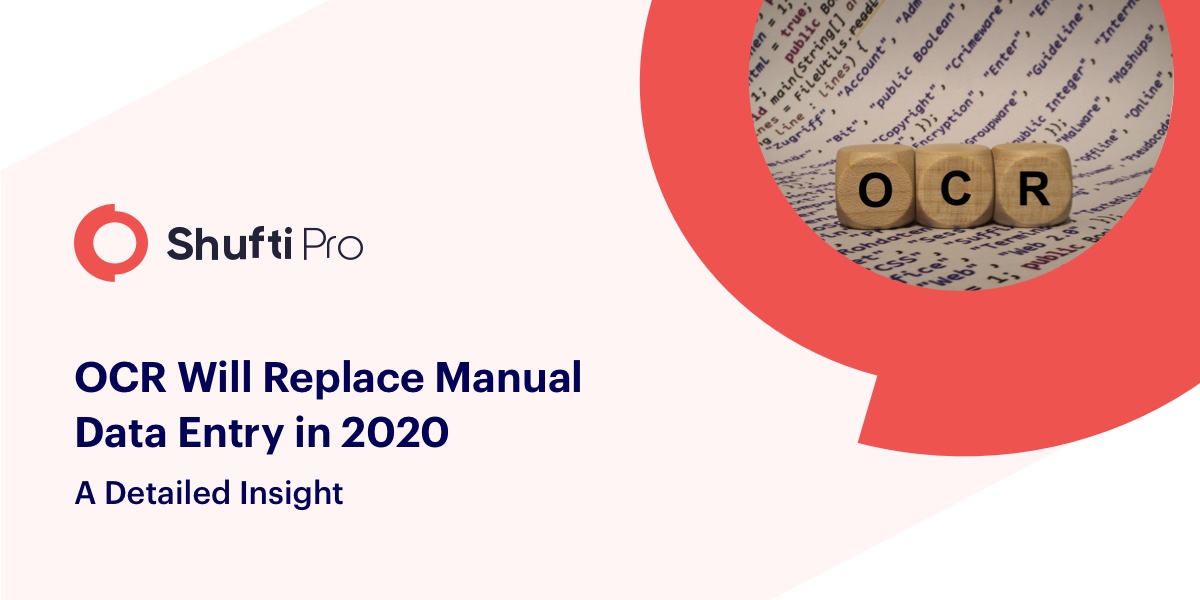
Living in the data-driven world, there is a huge demand for storing data from printed or handwritten documents to computer storage disk to reutilize and process the data for multiple business operations. Document processing is an essential part of business operations, yet it consumes quite a valuable time of the user. Data entry has always been a hectic job and organizations are striving to discover new ways to automate it. Whatever the solution is, it must have to be efficient enough to accurately fetch and populate data, especially in the case of financial and identity documents.
OCR technology has been invented to address this issue effectively. The automated data entry solutions are accurately replacing the work done by data entry operators. Originally created to replace the data entry process and with time OCR technology has come a long way.
What is OCR Technology?
Optical Character Recognition – commonly known as OCR – is a technology used for the mechanical or electronic conversion of images (scanned or printed documents, etc.) into machine-encoded text. OCR, widely used as a way of information extraction from passports, invoices, identity documents, bank statements, etc., is a prevalent method for digitizing the image text. The extracted information may be electronically displayed, edited and stored, which can be further used for cognitive computing and machine learning. Simply put, OCR technology is used to read and extract the data from image documents and then further used for pattern recognition.
AI-Powered OCR Technology – The Working
The old-school OCR technology wasn’t fully automated and couldn’t operate properly with manual supervision. The proper functioning required strict rules and templates. Still, these solutions couldn’t process context and had no self-regulating mechanisms; hence, making manual guidance mandatory.
The traditional OCR works quite well when it deals with documents whose formats and templates were pre-loaded into the system. However, this comes with a significant problem of flexibility. It means for every type of document a new template model has to be designed and loaded into the system. This is quite time-consuming and costly processes similar to the manual data population.
Therefore, artificial intelligence is being incorporated into OCR to come up with a flexible and reliable automated process. The working mechanism of such systems is based on three major stages and requires no manual interference.
1. Pre-Processing
For successful character recognition, the images are preprocessed using different techniques:
De-skew and Despeckle
Documents need to be aligned properly without any spots or crumpled/folded edges in order to accurately extract information or data from it. De-skew technique tilts the document a few degrees to make it perfectly horizontal and vertical. Additionally, in this process, the edges of documents are smoothened and spots are removed.
Binarisation
Binarisation is the technique of converting the colored image to binary image, i.e. grey-scale (black and white). It is necessary as most OCR algorithms work on binary images for the sake of simplicity. It also influences the recognition quality to a significant extent for making careful decisions on the provided input.
Layout Analysis and Line Removal
It identifies the columns, paragraphs and distinct blocks, filtering out non-glyph boxes and lines, particularly in the case of tables or multicolumn layouts. This aspect of pre-processing enables OCR technology to identify text and data written in the form of columns so that the data extraction is thorough and no text is left un-scanned.
Script Recognition
In multilingual documents, the scripts may change at the level of words, which makes the identification of scripts necessary before the character recognition process. It helps in enhancing the data extraction as the appropriate OCR parameters can be invoked for the specific script.
Character Isolation
Multiple characters combined due to image artifacts are separated for character OCR. This process is also known as “segmentation”. Segmentation of fixed-pitch fonts is easier and can be succeeded by placing the image on the grid. Due to the uniformity of the white spaces between characters, vertical lines least intersect black areas of characters. However, for proportional fonts, the more advanced approach is required because of the presence of irregular white spaces.
2. Character Recognition
Character Recognition works in two ways:
Pattern Recognition
Pattern Recognition works on the “Matrix Matching” algorithm, which compares the image to a stored glyph, pixel-by-pixel. It relies on the correct isolation of the input glyph stored accurately as per a similar font and scale. This technique works flawlessly for the typewritten document in the same font.
Feature Extraction
Pattern recognition can be ambiguous in the case of multilingual documents. Instead of identifying the character as a whole, feature extraction identifies the individual components of a particular character by decomposing it into “features” e.g. lines, line intersections, closed loops, line directions, etc.
These features are then compared with the abstract vector-like representation of the character which makes the entire character recognition process computationally efficient. This whole comparison process is done using the “k-nearest neighbor algorithm” which decides the nearest match. For example, the alphabet “A” has three individual components; 2 diagonal lines “ / ” “ \ ” and 1 horizontal line “ _
3. Automated Form Population
Form populate can be seen as an automated data entry process. The stored data in the memory from ‘Pre-processing’ and ‘Recognition’ steps, is populated in the requisite fields of the verification form; saving the time of end-user.
To increase the OCR accuracy for the document, the output is constrained to some post-processing techniques. “Near neighbor analysis” is one such technique, which uses the concept of co-occurrence frequencies to correct errors and identify certain words that should be written together. For example, “Washington DOC” is always written as “Washington D.C.
OCR as a whole in identity verification
As the world is moving towards digitization, the identity verification market is booming. Whether it’s about KYC requirement or fraud prevention, identity verification is becoming a significant part of every business. Gone are the days when customers used to verify their identities by physically visiting the organizations and showing their ID cards. Now the digital platforms require digital verification and document verification is an essential part of ID authentication.
In online verification, the system requires customers to upload their ID documents to get their identity verified. Most of the time user has to fill the online form himself which eventually takes a user precious time. In the race of user experience, the verification process has to be quick and seamless with the minimum manual interaction. That’s how ID verification solutions are incorporating OCR technology to make customer experience frictionless.
Shufti’s ID verification service is an example of such solutions for integrating OCR technology. The instant capture feature built on OCR quickly captures and extracts information from the identity documents, keeping customers from the mundane task of data entry. Moreover, the data extraction is applicable for both printed and handwritten documents. The whole process from data extraction to form population doesn’t take more than 2 seconds making the process fully automated and accurate.