Face Spoofing & Liveness Bypass: The Real Threat to Facial Recognition
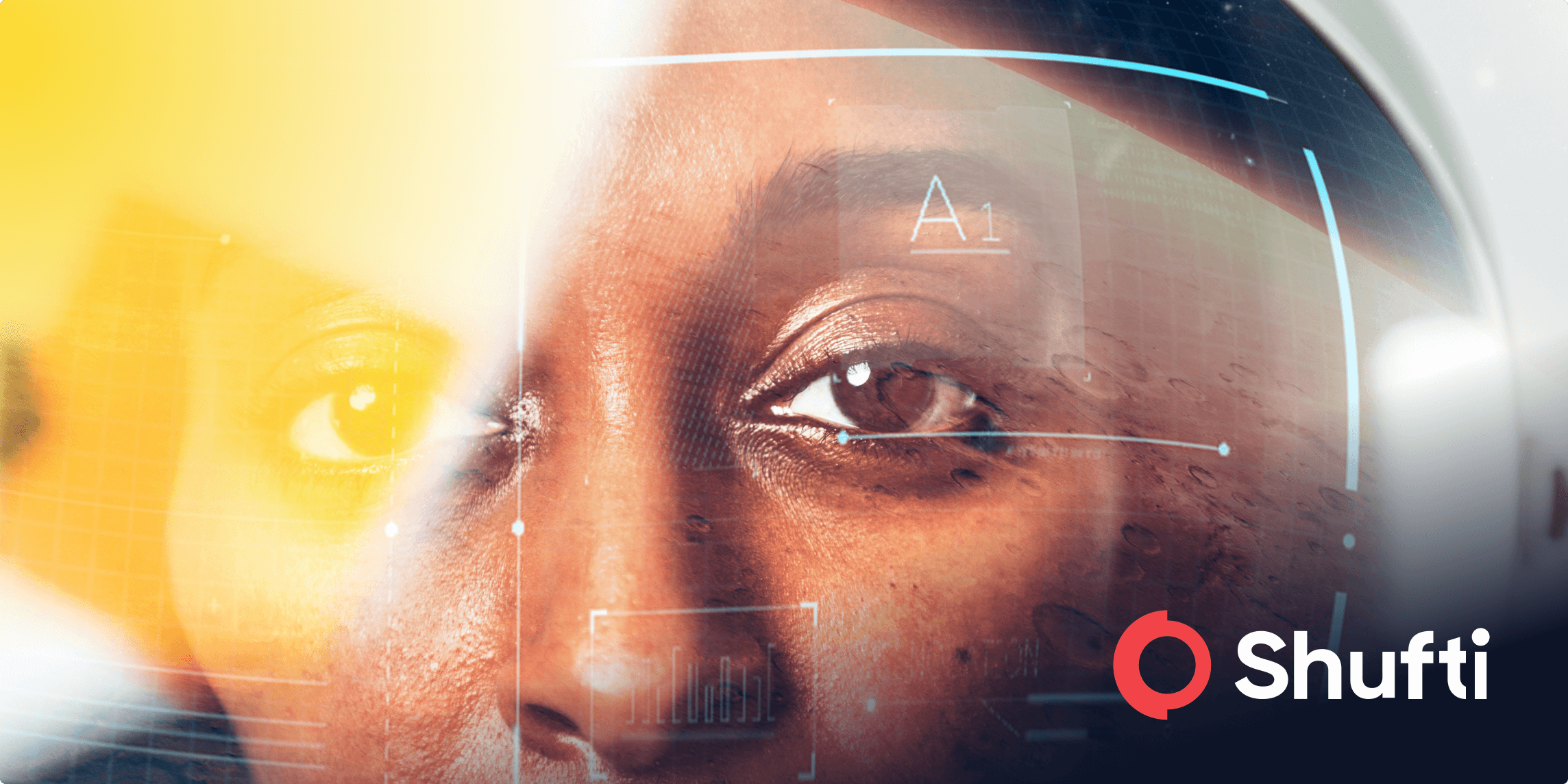
With the introduction of facial recognition, authentication has never been faster or more convenient. Now instead of passwords and ID cards, all you need is a quick glance. However, this convenience comes at a cost because as the technology spreads so do the methods to exploit it.
Between spoofing attacks utilizing masks and images to deepfake-fueled impersonations, fraudsters are always evolving. If your system isn’t ready to detect fakes and confirm real human liveness, it’s already vulnerable.
How Criminals Exploit Face Verification
1. Face Spoofing (Presentation Attacks)
Spoofing occurs when fraudsters work to trick facial recognition systems using artificial visuals. The two main types:
- 2D Attacks: Printed photos, screen replays, or videos shown to a camera
- 3D Attacks: Silicone masks, 3-D printed heads, or robotic replicas that mimic facial facial movements
While 2D attacks have historically been more common, 3D attacks have become more common with advancements in printing and robotics. Deepfakes add even more complexity with high-quality synthetic videos that can fool the human eye.
2. Liveness Bypass (System Exploits)
Bypass attacks focus on exploiting the flow of information between the device to the facial recognition technology by:
- Injecting pre-recorded videos using compromised devices
- Intercepting or replacing biometric data in transit
- Hacking backend serves to manipulate results
These methods tend to utilize malware, deepfake tools, or unsecured data channels.
How to Stop Face Spoofing and Bypass Attacks
- 3D Liveness Detection
Systems must measure depth, facial texture, and microexpressions, such as blinking or muscle movements, to ensure the person on camera is live and present.
- Multi-Factor Authentication (MFA)
Facial recognition should not be the only line of defense so a second layer, be that a password, device based check, or behavioral data, should be added.
- Secure Data Handling
Strong end-to-end encryption for biometric data is crucial during transmission to prevent replay and man-in-the-middle attacks.
- Anti-Spoofing AI
Machine learning is able to detect various spoofing techniques including photos, masks, and deepfakes through pixel-level analysis.
- Real-Time Monitoring
Unusual login patterns, behavioral changes, and system anomalies are all signals that an attack is in progress.
- Frequent Updates & Testing
Regular system updates and penetration tests help find security vulnerabilities before attackers do.
Choosing a Secure Liveness Solution
Not all liveness detection solutions are created equal and the gulf between them can be significant. Many basic systems are fooled by something as simple as a photo, video, or screen replay. That’s why it’s very important to conduct real-world testing before deployment.
Some ways to test a liveness solution include:
- Place a static image, either on paper or on a screen, in front of the camera. Any worthwhile system will recognize the lack of depth and facial movement.
- Try to authenticate with closed eyes and without facial muscle movements. Proper liveness checks analyze blinking, micro-movements, and skin texture changes.
- Test deepfakes and 3D masks (this is especially important for industries that face significant fraud threats). Effective systems are able to detect incorrect light reflections, a lack of skin elasticity, and shallow facial depth.
In addition to performance, it’s important to analyze the security protocols in place, such as:
- Does the solution employ end-to-end encryption for the collected biometric data?
- Is it certified under standards like ISO/IEC 30107-3 for presentation attack detection?
- Have they published independent audits that prove their system’s resilience against spoofing?
A good liveness solution should be able to combine technical strength with transparency and regulatory alignment.
How Shufti Fights Fraud
Shufti’s facial verification system is designed to handle real-world threats (not just engineered scenarios). It utilizes advanced AI, machine learning, and biometric science to deliver both accuracy and security.
- 3D Liveness Detection
Digs beyond surface-level checks to capture facial depth, motion, and spatial features in order to verify that a person is present (and not wearing a 2D or 3D mask).
- Microexpression Analysis
Detects if subtle, involuntary facial movements like blinking, eye twitches, or muscle tension are present. With current technology, these cues are virtually impossible to replicate in masks or deepfakes.
- AI Mapping
Leverages deep neural networks to identify and match facial features with high accuracy, even in suboptimal lighting, changing angles, or minor facial changes.
- Flexible, Scalable Integration
Easily integrates into existing workflows across web, mobile, and desktop environments so while devices might change, the quality remains.
- Proven Compliance and Security
Shufti’s Liveness Detection systems are fully compliant with worldwide data regulations such as GDPR, CCPA, and ISO/IEC 30107-3. It’s also regularly tested against evolving spoofing techniques, including deepfakes, synthetic identities, and advanced 3D printed masks.
Final Thoughts
Facial recognition has helped redefine how we authenticate, however it can only be as strong as the systems build to protect it. As spoofing tactics and liveness bypasses become more advanced, organizations must grow from basic face checks to truly intelligent, secure solutions.
The way to do so is clear: combine advanced liveness detection, AI-powered fraud prevention, and rigorous testing standards. Whether you’re verifying users, securing access, or preventing digital impersonation, the right technology makes all the difference.
With tools like Shufti’s AI-driven face verification system, businesses can continue to confidently embrace facial recognition (without inviting modern fraud).